Computational intelligence modeling of hyoscine drug solubility and solvent density in supercritical processing: gradient boosting, extra trees, and random forest models
Por um escritor misterioso
Descrição

Development of machine learning model and analysis study of drug

RFR Model: test and train data predictions. RFR Model: test and

Novel Solubility Prediction Models: Molecular Fingerprints and

Computational intelligence modeling of nanomedicine preparation
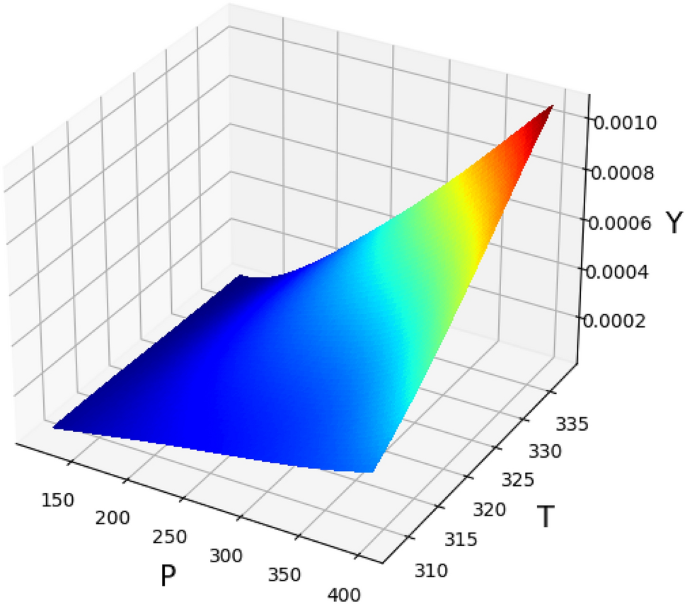
Computational simulation and target prediction studies of

Evaluation of Deep Learning Architectures for Aqueous Solubility

Evaluation methodology based on k-fold crossvalidation.
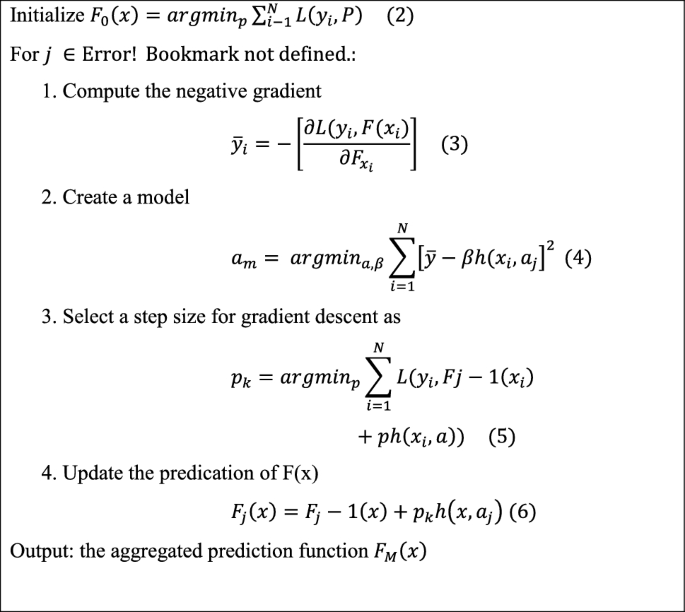
Design of predictive model to optimize the solubility of Oxaprozin
Cluster-Based Regression Model for Predicting Aqueous Solubility
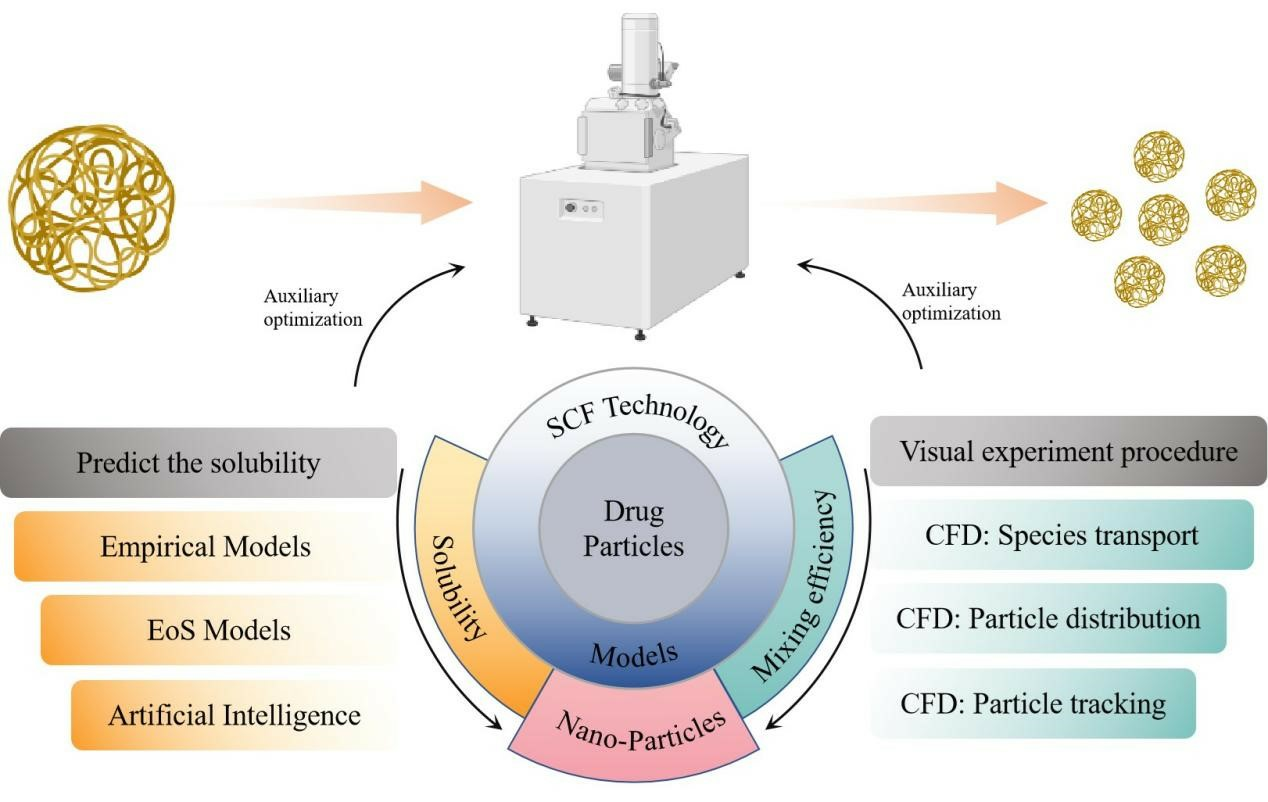
Bioengineering, Free Full-Text

Data distribution, P (pressure), T (temperature), and Y

Three-dimensional illustration of inputs/outputs (GBRT Model

Replacement solvent suggestions for procedures involving benzoic

PDF) Computational intelligence modeling of hyoscine drug
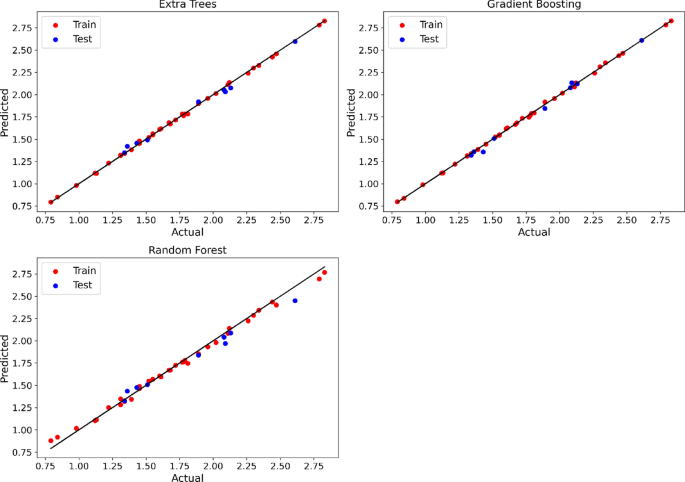
Computational intelligence modeling of hyoscine drug solubility
de
por adulto (o preço varia de acordo com o tamanho do grupo)